A/B Testing is an experimental approach to comparing two or more versions of a particular thing. The end goal is to find out if there is a difference in performance and confirm which one actually performs better.
In marketing, advertising, and product management, it’s common to A/B test things such as email subject lines, website copy, landing pages, call-to-action (CTA) buttons, product features, or user interfaces.
To add more context, let’s look at a dilemma that Obama’s campaign team faced in 2007. The team was struggling to convert website visitors into subscribers, so they decided to A/B test three new variants of the CTA button: “Learn More,” “Join Us Now,” and “Sign Up Now.” Their test results showed that “Learn More” garnered 18.6% more signups per visitor than the default “Sign Up.”
In another scenario, the team believed that a video of Obama speaking at a rally would perform better than any still image, but after A/B testing, the results showed that the video performed 30% worse than the image.
Essentially, A/B testing is an agile approach that helps teams make decisions backed by data rather than instincts. Doing this improves conversion rates by ensuring they launch a more successful version of their campaign or product.
How does A/B Testing Work?
Like any standard experiment, A/B testing is based on a hypothesis. The hypothesis should be focused on one specific problem and be testable using a clearly defined success metric, which can complement insights gained from heuristics in business strategy.
Consider the hypothesis, “Shortening the registration form on our landing page will increase sign-up rates.” This could mean that the current registration form has three form fields and is believed to be less effective than a form with one form field.
The original version of the element you want to test—a form with three form fields—is labeled “control” and the modified version—a form with one form field—is labeled “treatment” or “variant”.
The test works by showing a fraction of users the different versions and then determining which one influenced your success metric the most. If the variant leads to more sign-ups, it will displace the original. But if not, it will be terminated and most users will never get to see it.
The standard practice is to test two versions or variants, but you can test more as long as you are only changing one element. In the case of the registration form, you can’t change both the number of form fields and the CTA button. You only change one element so that you can easily analyze the effect of that change.
Why should you use an A/B test?
Instead of guessing, marketers and business strategists use A/B testing to make data-driven decisions regarding how to optimize their website, content, or marketing campaigns to improve performance.
With A/B testing, you can:
- Test new ideas quickly
- Validate your hypothesis and get proof of what actually works for the audience
- Identify the most effective version of anything you want to launch
- Get direct unbiased feedback from actual users and customers
Anke Audenaert, in her Data Analytics Methods for Marketing course, shared that when she worked on the Yahoo search research team, they used A/B testing rigorously.
She also acknowledged that “small changes to the user experience on the search results pages could have a very big impact on the revenue that was generated from those search ads on those pages.”
Different Use Cases for A/B Testing
Here are a few use cases for A/B testing in marketing, advertising, and product management :
- Email marketing. Test different subject lines, email designs, or call-to-action buttons in email campaigns. Track metrics such as open rates, click-through rates, or revenue generated.
- Content marketing. Optimize your content by testing headlines, call-to-action links, or visual elements in blog posts or landing pages to understand what resonates best with your target audience and drives better results.
- Advertising and PPC Campaigns. Optimize ad copy, images, targeting criteria, or display formats to improve click-through rates or reduce the cost per acquisition (CPA).
- Mobile App Optimization. Test variations of feature placements, navigation flows, onboarding processes, or messaging to enhance user engagement, retention rates, or in-app conversions.
- UI/UX Design. Test different design elements, color schemes, layouts, navigation structures, or interaction patterns to improve usability, task completion rates, or user satisfaction.
Depending on your hypothesis, there are different A/B tests you can run to validate assumptions and influence your defined success metric.
- Call-to-Action (CTA) test. This involves testing different variations of CTA buttons or links to determine which CTA design, wording, color, size, or placement drives higher click-through rates.
- Messaging test. This is focused on testing variations of your marketing messaging or written content, such as headlines, product descriptions, taglines, email subject lines, and ad copy. The objective is to determine which variation resonates better with the target audience or drives higher engagement.
- Pricing test. This compares different pricing models or discount strategies to identify the most effective pricing for a product or service. Variations may include changes in pricing tiers, discount structures, packaging options, or subscription models.
- Landing page test. Focuses specifically on testing variations in layout, headline, social proof elements, or content. For example, using a video that explains how a product works versus a video that features testimonials from customers.
- Navigation and user flow test. This is focused on optimizing the navigation and user flow within a website or application. You can test different versions of menus, navigation structures, or user paths to improve user engagement, reduce bounce rates, or enhance the overall user experience.
- Feature test. When you want to gauge user preferences, adoption rates, or satisfaction levels, you can use this to test different variations of product features or design elements.
- Checkout process test. Based on a success metric like “cart abandonment rate”, you can test variations in the number of steps, form fields, payment options, or shipping methods.
How to do an A/B Test?
- Define the hypothesis.
Formulate a hypothesis that specifies what you expect to achieve with the variations. Ryan Hewitt, senior business analyst at ASOS, recommends that your A/B test needs to have “a clear reason and a measurable outcome.”
He also recommends a format for writing testable hypotheses: We predict that <change> will significantly impact <KPI/user behavior> We will know this to be true when <measurable outcome>
- Choose the primary success metric.
This should directly align with your expected outcome and may be tied to revenue, conversion rates, customer acquisition, or user engagement. It should also be possible to accurately track and collect data related to the metric throughout the A/B testing process.
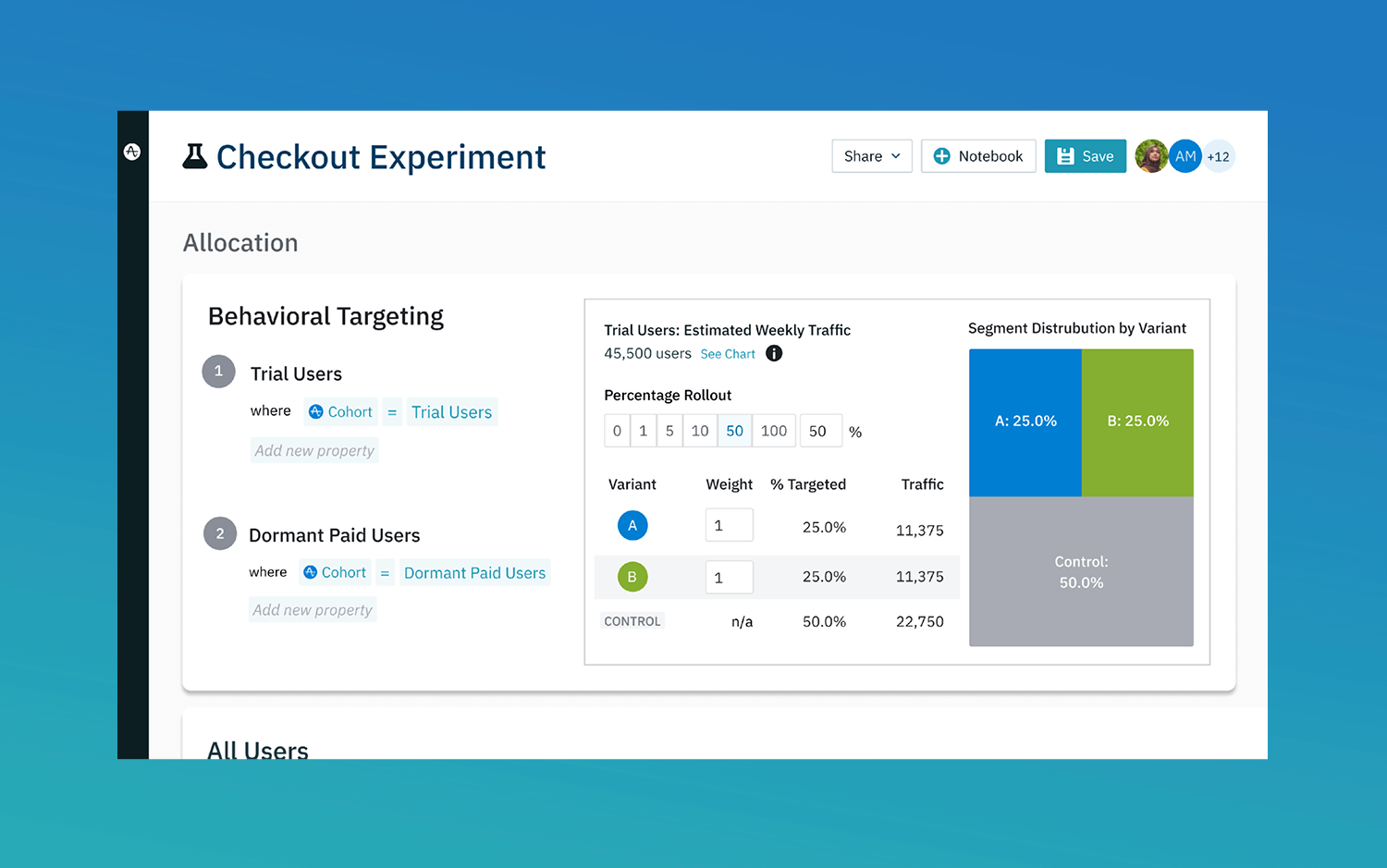
- Design the experiment
This includes three essential factors:
- The two distinct versions of the element you’re testing.
- The portion of your audience that will participate in this experiment. A good rule of thumb is to collect data from at least 100 users. If you don’t have enough data, the results of an A/B test may not show a clear difference. Make sure you can identify visitors to your website as this will help with segmentation.
- The duration of the test, i.e. how long the test will run. The communications team at Adobe recommends it should be “long enough for you to gather meaningful data.”
- Run the experiment
At this point, you have to choose a tool you will use to run this experiment. Most of the top marketing and digital analytics software have built-in A/B testing features. So before you start scouring the internet for a new tool, look through the platform you currently use to see if it has A/B testing capabilities.
Different tools include Amplitude Experiment, AB Tasty, Statsig, or Hubspot’s A/B Testing Kit. For each of these tools, look out for segmentation and targeting capabilities, statistical analysis and reporting features, and integration with other tools.
- Analyze results
Most A/B testing tools will do the calculations, analysis, and implementation for you, but you should review the results to compare the performance of the two versions and gather insights.
Determine the level of improvement based on your success metric and the significance of the differences between the original and modified versions.

Conclusion
Remember, the specific type of A/B test will depend on the goals, elements, and metrics you are focusing on. A well-designed A/B test involves having clear variations, a sufficient sample size, and a proper measurement plan to gather meaningful insights and make informed decisions.